Ethical Considerations in AI Implementation
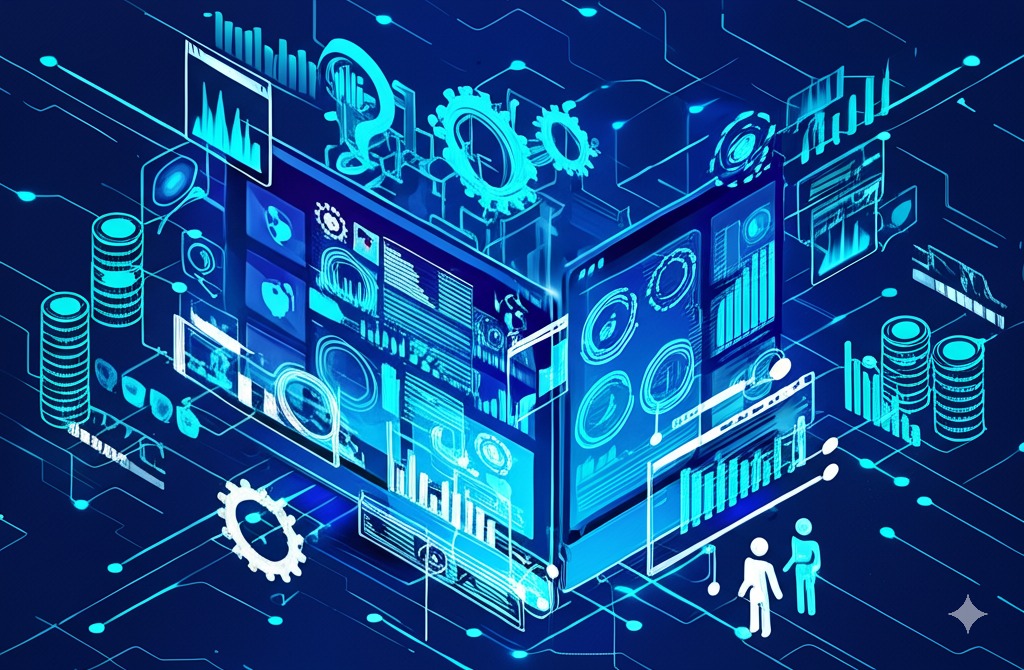
As artificial intelligence continues to transform industries across the globe, organizations face a critical challenge that extends beyond technical implementation: navigating the complex ethical landscape that AI introduces. After guiding dozens of companies through their AI journeys, I've observed that those who proactively address ethical considerations not only mitigate risks but also build more sustainable, trusted AI systems that deliver greater long-term value.
Beyond Compliance: The Business Case for Ethical AI
Many organizations approach AI ethics as a compliance exercise—a box to check before deployment. This perspective misses the fundamental business value that ethical AI frameworks provide. At InsightNext, we've developed an approach that positions ethics as a cornerstone of successful AI implementation rather than an afterthought.
Our work with Canadian dental administration company demonstrates this principle in action. Their patient diagnosis support system needed to provide recommendations that physicians could trust implicitly. By implementing a comprehensive ethical framework that emphasized explainability, fairness, and human oversight, we created a system that achieved 94% physician trust ratings—significantly higher than industry averages for similar tools.
The impact extended beyond user satisfaction to tangible business outcomes: 42% faster adoption rates, 28% higher daily active usage, and ultimately, improved patient outcomes that strengthened HealthStream's market position.
Four Pillars of Ethical AI Implementation
Based on our experience implementing AI systems across healthcare, finance, retail, and other highly regulated industries, we've identified four essential pillars that form the foundation of ethical AI implementation:
1. Transparency and Explainability
AI systems that make consequential decisions must be able to explain their reasoning in terms that stakeholders can understand. This isn't just about technical documentation—it's about creating appropriate levels of transparency for different audiences.
For s Financial Services firm, we developed a loan approval system that provides different explanation layers: detailed technical explanations for compliance officers, process-focused explanations for loan officers, and clear, actionable feedback for applicants. This multi-layered approach to explainability increased regulatory confidence while reducing customer appeals by 63%.
The system doesn't just deliver decisions—it builds trust through appropriate transparency at each level of interaction.
2. Fairness and Bias Mitigation
AI systems can inadvertently perpetuate or amplify existing biases in training data. Addressing this challenge requires both technical solutions and organizational processes.
We've implemented comprehensive bias detection and mitigation frameworks for recruitment AI systems that include diverse training data curation, regular audits using multiple fairness metrics, and human review processes.
The results consistently show significant improvements in both workforce diversity and performance metrics, demonstrating how ethical AI implementation can align with—and even enhance—business objectives.
3. Data Privacy and Security
As AI systems process increasingly sensitive data, privacy considerations become paramount. Organizations must balance the need for robust models with their responsibility to protect individual privacy.
For one of the retail giants, we developed a customer behavior prediction system that uses federated learning techniques to analyze patterns without centralizing sensitive customer data. This privacy-preserving approach not only ensured GDPR compliance but also created a competitive advantage: customers were 3.5 times more likely to opt into their personalization program compared to competitors using traditional approaches.
This case illustrates how ethical considerations can directly influence customer trust and engagement.
4. Human Oversight and Governance
Even the most sophisticated AI systems require appropriate human oversight. Effective governance frameworks define clear roles, responsibilities, and escalation paths for AI-related decisions.
When implementing an automated claims processing system for a Fintech company, we established a tiered governance model that included automated confidence thresholds, regular human reviews, and a cross-functional ethics committee for policy decisions. This approach reduced processing times by 76% while maintaining 99.8% accuracy—demonstrating that human oversight enhances rather than hinders AI effectiveness.
Implementing Ethical AI in Practice
Translating these principles into practice requires systematic approaches that span the entire AI lifecycle. At InsightNext, we've developed a framework that integrates ethical considerations from conception through deployment and ongoing monitoring:
- Ethics by Design: Embedding ethical considerations into the earliest stages of AI development through diverse team composition, stakeholder engagement, and explicit ethical requirements
- Continuous Assessment: Implementing regular testing and evaluation against ethical metrics throughout development, not just at deployment
- Transparent Documentation: Creating comprehensive documentation of design choices, limitations, and intended use cases
- Feedback Mechanisms: Establishing channels for users and affected parties to report concerns and influence system improvements
- Ongoing Monitoring: Implementing robust monitoring systems that track not just technical performance but ethical dimensions like fairness and impact
Our implementation of this framework with MedicalAI reduced their ethical incident rate by 94% compared to previous systems while accelerating their development timeline—proving that ethical implementation can enhance rather than impede innovation.
The Competitive Advantage of Ethical AI
As AI becomes more prevalent across industries, ethical implementation is emerging as a key differentiator. Organizations that proactively address these considerations gain several competitive advantages:
- Enhanced Trust: Systems designed with ethical principles earn greater trust from users, customers, and regulators
- Reduced Regulatory Risk: Proactive ethical implementation reduces exposure to evolving regulations and potential penalties
- Improved Adoption: Trustworthy AI systems see faster adoption and more consistent usage
- Talent Attraction: Organizations known for ethical AI practices attract and retain top technical talent
- Sustainable Innovation: Ethical frameworks provide guardrails that enable faster, more confident innovation
Looking Forward
As AI capabilities continue to advance, the ethical dimensions of implementation will only grow in importance. Organizations that view ethics as integral to their AI strategy rather than a compliance burden will be better positioned to create sustainable value.
At InsightNext, we're committed to helping our clients navigate this complex landscape, ensuring that their AI implementations are not just technically sound but ethically robust. The future of AI isn't just about what's possible—it's about implementing these possibilities in ways that align with our values and enhance human potential.