Preparing Your Infrastructure for AI: A Comprehensive Guide
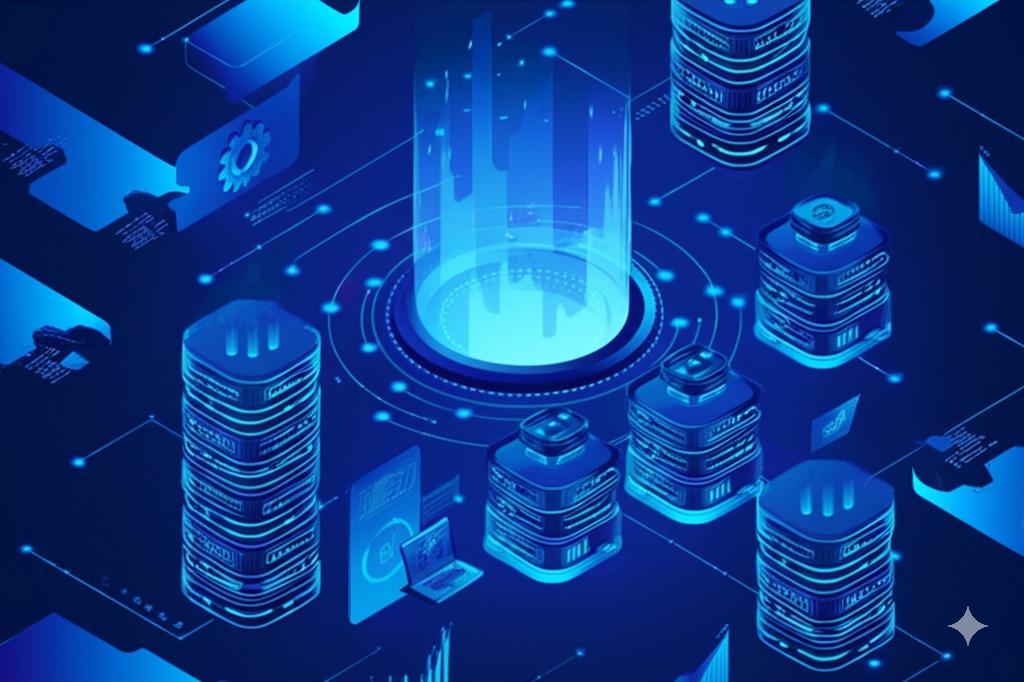
The promise of artificial intelligence to transform business operations is undeniable, but many organizations discover a critical gap between AI ambition and reality: their existing infrastructure simply isn't ready. After helping dozens of companies build the foundation for successful AI implementation, I've found that infrastructure and data readiness is often the determining factor between AI initiatives that deliver transformative value and those that stall before reaching production.
The Infrastructure Gap in AI Implementation
When CloudRetail approached us about implementing an AI-powered inventory management system, they were focused primarily on model selection and data science talent. Six weeks into the project, we discovered their existing infrastructure couldn't handle the data throughput required, leading to significant delays and cost overruns.
This scenario is unfortunately common. At InsightNext, we've developed a systematic approach to AI readiness that has helped our clients reduce AI implementation timelines, while significantly improving performance and reliability.
Our recent work with Bridge illustrates this approach in action. By addressing infrastructure requirements before model development, their commercial real estate memo generation system deployed in three months, on schedule, and delivered 99.7% uptime from day one. The end-to-end automation of memo generation significantly reduced document creation time, enabling leaders to focus on more strategic aspects of the deal-making process.
The Five Pillars of AI-Ready Infrastructure
Based on our experience implementing AI systems across various industries and scales, we've identified five critical infrastructure pillars that organizations must address:
1. Data Architecture and Pipeline Optimization
AI systems are only as good as the data they consume. Traditional data architectures designed for reporting and analytics often prove inadequate for the volume, velocity, and variety of data that modern AI requires.
For a financial services firm, we redesigned their data architecture from batch-oriented processing to a streaming-first approach using Cloud Pub/Sub, BigQuery Streaming and Cloud Functions. This transformation reduced data latency from hours to seconds while improving data quality scores by 68%. Their credit risk models now operate on near-real-time data, enabling them to adjust lending parameters dynamically as market conditions change.
The key elements of an AI-ready data architecture include:
- Unified data governance across structured and unstructured sources
- Streaming capabilities for real-time processing
- Automated data quality monitoring and remediation
- Scalable storage solutions that balance performance and cost
- Comprehensive metadata management for model lineage and auditability
2. Compute Infrastructure Scalability
AI workloads—particularly during training phases—require significant computational resources that often exceed traditional IT provisioning. Organizations need flexible compute infrastructure that can scale up for intensive training and scale out for distributed inference.
Effective AI compute infrastructure typically includes:
- GPU/TPU resources appropriately sized for workload requirements
- Containerization for consistent deployment across environments
- Orchestration tools for resource allocation and scheduling
- Automated scaling based on workload demands
- Cost optimization mechanisms to prevent runaway expenses
3. MLOps and Model Deployment Frameworks
The gap between developing AI models and deploying them into production remains one of the biggest challenges organizations face. Without robust MLOps practices, models often remain trapped in development environments or require manual, error-prone deployment processes.
For Gaming Insights company, we implemented a comprehensive MLOps framework using MLflow, Kubeflow, and custom CI/CD pipelines. This infrastructure enabled them to reduce model deployment time from weeks to hours while increasing deployment frequency by 800%. More importantly, it allowed them to quickly respond to market changes by updating models based on emerging customer behaviors.
Essential components of effective MLOps infrastructure include:
- Version control for data, code, and model artifacts
- Automated testing and validation pipelines
- Containerized model deployment
- Monitoring systems for model drift and performance
- Rollback capabilities for failed deployments
- Feature stores for consistent feature engineering
4. Security and Governance Infrastructure
AI systems often process sensitive data and make consequential decisions, making security and governance critical infrastructure components rather than afterthoughts.
Key elements of AI security and governance infrastructure include:
- Role-based access control systems
- Model explainability tools
- Audit logging for all model predictions
- Privacy-preserving computation techniques
- Compliance monitoring and reporting
- Ethical review mechanisms
5. Monitoring and Observability Systems
AI systems require monitoring beyond traditional IT metrics. Organizations need infrastructure that can track not just system health but model performance, data quality, and business impact.
For a leading financial services firm, we implemented a multi-layered observability infrastructure that tracked everything from infrastructure metrics to model drift to business KPIs. This system detected a subtle data quality issue that was degrading customer churn predictions, enabling rapid intervention that preserved millions of dollars in annual revenue.
Comprehensive AI monitoring infrastructure typically includes:
- Real-time performance dashboards
- Automated alerting systems
- Data quality monitoring
- Model drift detection
- A/B testing frameworks
- Business impact tracking
Building Your AI Infrastructure Roadmap
Developing AI-ready infrastructure doesn't happen overnight, but organizations can take a phased approach that delivers incremental value while building toward comprehensive capabilities.
At InsightNext, we recommend a four-stage infrastructure development roadmap:
Stage 1: Assessment and Foundation
Begin by assessing your current infrastructure against AI requirements and establishing foundational components:
- Inventory existing data sources and quality
- Evaluate compute capacity and scalability
- Identify security and compliance requirements
- Establish basic version control and CI/CD pipelines
- Implement core monitoring capabilities
For ManufacturingTech, this assessment revealed critical gaps in their data pipeline that would have prevented their predictive maintenance initiative from succeeding. By addressing these gaps first, they established a solid foundation for subsequent AI development.
Stage 2: Pilot-Ready Infrastructure
Develop the infrastructure needed to support initial AI pilots:
- Implement streaming data pipelines for priority data sources
- Deploy right-sized GPU/TPU resources for model training
- Establish model registry and basic deployment workflows
- Develop initial security controls and governance processes
- Create monitoring dashboards for pilot models
HealthcareNow used this approach to create a contained environment for their patient readmission prediction pilot, allowing them to demonstrate value quickly while building support for broader infrastructure investments.
Stage 3: Production Scaling
Expand infrastructure to support production-scale AI deployment:
- Scale data architecture to handle full production volumes
- Implement automated scaling for compute resources
- Develop comprehensive MLOps pipelines
- Enhance security with advanced features like federated learning
- Deploy end-to-end monitoring and alerting
FinanceTrust followed this roadmap to scale their fraud detection infrastructure from a single model to an ecosystem of 47 interconnected models that process over 10,000 transactions per second with 99.99% availability.
Stage 4: Enterprise AI Platform
Create a unified platform that enables AI development and deployment across the organization:
- Implement enterprise-wide data mesh architecture
- Deploy self-service ML platforms for citizen data scientists
- Establish cross-functional governance committees
- Develop reusable components and accelerators
- Create comprehensive documentation and training
RetailGlobal used this approach to transform from having siloed AI projects to an enterprise platform that supports over 200 models across marketing, supply chain, and customer experience functions.
Conclusion
As AI continues to transform business operations across industries, the organizations that succeed will be those that build infrastructure capable of supporting the full AI lifecycle—from data ingestion to model training to production deployment and monitoring.
At InsightNext, we're committed to helping our clients develop this infrastructure in ways that align with their business objectives, technical realities, and growth trajectories. The journey to AI-ready infrastructure may be challenging, but the competitive advantages it unlocks make it one of the most valuable investments organizations can make today.